Synthetic data offers unparalleled research agility, spanning quantitative and qualitative methodologies. However, as with any AI deployment, reliability is a crucial consideration. This article explores the technology driving synthetic data providers, its various use cases, and essential safeguards for effective management at scale.
While we are certain that there is a tremendous opportunity with synthetic data, we acknowledge the skepticism surrounding it. It is only natural to question while navigating unfamiliar territory. Can we trust synthetic data? How will it credibly replace human insights? Will it take jobs away from researchers?
We hear you, and we are here to address these challenges head-on.
But before we get into all the good stuff, let’s take a step back to reflect on the previous research revolutions that have led us to where we are today.
History of Data Collection
The evolution of data collection has been remarkable, adapting to technological advancements and societal demands. With each milestone, the market research industry has expanded, making insights more accessible and, in turn, easing business decision-making.
Take a moment with us as we run back through the history of data collection.
1930s - From face to face interviews to the telephone sampling revolution
Does the name Gallup ring a bell?
Let’s transport to the economically and politically turbulent American society of the late 1930s.
Against the backdrop of the Great Depression, Americans found unity through new forms of mass communication, which served as channels of sharing public opinion. Whether through radio broadcasts, magazines, newspapers, or films, these mediums characterized and reflected the prevailing sentiments of the time.
Meanwhile, George Gallup, a journalism professor and marketing expert, began asserting his life to understanding and reporting “the will of the people.” Tapping into the potential of these expansive platforms to study public opinion, Gallup conducted national surveys via face-to-face interviews to gain insights into emerging trends. Grounded in principles of objectivity and the pursuit of unbiased data, he refused to entertain sponsored or paid polling, prioritizing authentic findings. Decades later, during the 1980s, phone interviews became the leading collection method as most American households had become equipped with telephones.
Now synonymous with public opinion research, Gallup’s breakthrough came in 1936, when he accurately predicted Franklin Roosevelt’s victory over Alfred Landon in the presidential election. His non-partisan polling methodologies based on phone sampling proved to be more representative and precise, cementing his legacy as an American pioneer of opinion polling. By laying the groundwork for consumer research, Gallup cultivated a profitable industry and established himself as a household name.
2000s - Online sampling revolution
At the turn of the century, data collection methods underwent a major transformation. While the 1930s saw the rise of phone interviews as a fresh way to gather insights from people, the 2000s brought about a seismic shift with advancements in technology and internet usage. This paved the way for a game-changing method: online surveys. Suddenly, public opinion, consumer preferences, and social trends could be tapped into through the click of a mouse. With the internet at our fingertips, researchers found themselves with access to a wider, more diverse pool of respondents. Online forums suddenly made data collection faster and more cost-effective, and they granted a degree of anonymity for participants. The chart below reveals Pew Research Center’s analysis of public polling transformations in the 21st century. The advent of online polling democratizing research efforts and driving industry growth is clear.
.png)
Today - AI & the future of data collection
From Gallup’s trailblazing phone interviews to the emergence of online surveys in the early 21st century, we have witnessed monumental leaps in data collection methodologies. But now, we stand on the brink of another groundbreaking frontier that explores the endless potential of generative AI and synthetic data.
Predicted by Gartner to surpass real data in AI models by 2030, synthetic data is taking over the market research space. Able to streamline research processes and enable researchers the capacity to dive deeper into complex segments, synthetic data reveals insights previously out of reach. The substantial business benefits it offers also bring forth inherent risks. Is it reliable? Is it replacing human roles? Is the large-scale deployment of AI ethically sound?
Researchers around the world are experimenting with innovative technologies and challenging their capabilities.
In the qualitative world, virtual agents and audiences are streamlining insights at an unprecedented pace, with dozens of new vendors in the space. In the quantitative world, where statistical guarantees are in place, Fairgen is among the very first to provide predictive synthetic respondents with statistical guarantees. In its foundational white paper, it has shown that it can generate synthetic sample boosters that are, on average, worth three times the amount of real respondents.
The mixed sentiments about synthetic data are loud and will continue to echo in our industry in the next years.
Experienced in the marketing field, Dr. Mark Ritson validates that promising potential is more often confirmed than contradicted, asserting that “synthetic data is suddenly making very real ripples.” Meanwhile, Kantar suggests that synthetic samples generated by LLMs are deemed unable to “meet the brief,” as they lack variation and nuance and exhibit biases. Although the hesitation is underscored in discussions concerning synthetic data, this space is closely monitored as the next technological innovation.
Today, nearly a century later, our team at Fairgen is still on the mission Gallup started, boosting the research into the will of the people, retaining the principles of scientific rigor and statistical guarantees.
What is Synthetic Data?
It’s time we truly grasp the concept of synthetic data.
Synthetic data is information that is artificially manufactured as opposed to being collected or created by real-world events.
With the surge of computing power and generative AI technology, synthetic data is becoming increasingly popular across multiple industries, especially when data is scarce or sensitive to privacy concerns.
What are the most common types of algorithms behind synthetic data technology?
Explore the diverse branches of machine learning models for synthetic data generation and analysis.
- Generative models for tabular data are algorithms designed to understand and replicate the underlying structure and patterns within structured datasets. These models, ranging from classical statistical approaches to modern deep learning techniques like Variational Autoencoders (VAEs) and Generative Adversarial Networks (GANs), aim to capture the joint distribution of variables in the data. By learning the underlying probability distribution, generative models can then generate new samples that resemble the original data. This capability is particularly useful in scenarios where data generation is limited or expensive, enabling synthetic data generation for tasks like data augmentation, privacy-preserving data sharing, and simulation in various domains such as finance, healthcare, and marketing.
- Large Language Model, or LLM, is a form of artificial intelligence that generates human-like text stemming from vast amounts of training data, usually comprised of sources such as books, articles, or websites. LLMs have advanced natural language capabilities, and when users input queries, they can perform functions such as text generation, translation, summarization, or question-answering. LLMs have dominated the realm of language tasks, advancing the natural language processing field.
- RAG, or Retrieval-Augmented Generation, fuses retrieval-based and generative approaches for natural language processing frameworks. Models, such as LLMs, combine with a retrieval component, such as knowledge bases or corpus of documents, to generate informative responses. While retrieval-based methods have been traditionally used for tasks such as text summarization, question answering, and other text-based responses, they can provide external data as context for language models.
- Reinforcement Learning for Human Feedback, or RLHF, is a machine learning technique that leverages human feedback to optimize processes and boost self-learning capabilities. In this approach, an LLM agent interacts with a human user who provides feedback on its content or actions. This constructive feedback is then used to update the agent's strategy and information, training the software to make decisions that maximize outcomes and accuracy. The primary objective is to ensure that tasks are performed in ways that align closely with human goals, preferences, and real-world interactions.
How is synthetic data used for market research?
At Fairgen, we tend to split the impact of synthetic data in the research industry primarily into two categories: AI research assistants and synthetic respondents.
The first can help researchers throughout any study's workflow. Early birds and innovative insights professionals are already using AI assistants to help with survey ideation, generating hypotheses, testing scenarios, and planning complex research methodologies faster and more creatively than before.
Research agents are also becoming increasingly popular for managing interviews and virtual focus groups via chat, as well as other traditionally human-led research practices. Additionally, AI assistants are used to process insights at scale, whether via translation, summarization, data visualization, or other analysis functions.
While AI research assistants are accelerating the ideation, collection, and analysis of insights from real respondents, the rise of reliable generative AI technology is enabling the creation of near-real synthetic respondents. Synthetic samples can provide immense value, especially in cases where incident rates are low and when privacy issues arise.
In summary, AI and synthetic data are being swiftly adopted throughout the research lifecycle. Let’s dive into more specific use cases for quantitative and qualitative methodologies and explore the ways synthetic data is employed within each.
How can synthetic data be applied to qualitative research?
Imagine you are not just crunching numbers but delving into the details of human experiences, beliefs, and behaviors. That is qualitative research in a nutshell – digging deep into the why and how of things.
Now, let’s discuss how it can be applied to synthetic data. More often than not when conducting qualitative interviews, researchers are faced with high costs associated with sourcing respondents and running in-person interviews. This is where the solution of synthetic data swoops in. Synthetic data allows you to simulate interviews, observations, and interactions, generating a virtual domain that mirrors real-world scenarios– or data.
This synthetic approach offers researchers an opportunity to safeguard privacy and the flexibility to explore consumer preferences widely, analyze social trends, or unravel intricate human interactions. Combining qualitative research with synthetic data provides immense opportunities for investigation and understanding.
Research Agent
A research agent refers to an AI-powered system designed to conduct qualitative research by asking questions and customizing interactions based on user responses. They can operate at scale, in multiple languages, and 24/7. Modern virtual research agents can customize their interactions according to the interviewee’s answers and profile, enabling meaningful and deep insights without the direct oversight of a human.
Virtual Audiences
Leveraging large language models (LLMs), virtual audiences consist of simulated persona groups that can mimic real human responses after being pre-trained on existing data. When prompted by a researcher, this synthetic pool of personas can provide insights into trends, behaviors, and preferences.
Build Qualitative Research Setups
Synthetic data can also aid researchers in constructing qualitative research setups by providing a versatile platform for answering questions and designing research workflows. It allows researchers to simulate many scenarios, demographics, and behaviors to play around with specific research objectives and refine their approaches and methodologies in a controlled environment before implementation.
How can synthetic data be applied to quantitative research?
Quantitative research revolves around systematically collecting and analyzing numerical data to discover patterns, trends, and relationships within data sets. Unlike qualitative methods, “quant” studies aim to make predictions and provide statistical assurances that the samples studied accurately represent their population with a high level of confidence.
Its fusion with synthetic data occurs when the populations being studied are hard or impossible to reach or privacy regulations constrain research endeavors.
Synthetic data for quantitative research can serve as a valuable resource if its reliability can be proved and governed at scale. Let’s dive into a few use cases:
Data Augmentation:
Synthetic respondents can be generated to boost under-represented groups in quantitative studies with strong certainty. Fairgen, for instance, has proved that it can generate synthetic sample boosters that are statistically equivalent to three times the amount of real respondents.
Data Completion:
Researchers often encounter challenges around incomplete surveys and the need to analyze extensive tabular datasets containing numerous empty cells across multiple rows. These gaps may arise from respondents dropping out or skipping questions. However, modern AI systems can be trained in predicting and filling in these missing responses.
Market Segmentation:
In an increasingly diverse world, data segmentation enables researchers to understand specific consumer groups deeply. Unfortunately, thousands of niche studies are dropped yearly, as quotas are often not met on the granular level, negatively impacting the application of niche go-to-market strategies. Synthetic and predictive respondents, like those generated by Fairboost, can be a reliable solution to gain reliability on the niche level, as long as the niche groups are part of a larger study.
How can you scientifically validate synthetic respondents?
Employing synthetic data necessitates implementing measures to ensure quality and credibility. It is crucial to recognize that different synthetic data generation techniques come with varying levels of risk and require unique validation procedures.
Introducing the concept of the Blackbox is essential. In the realm of artificial intelligence, a Blackbox system entails an input entering the system and an output being released. The catch is that the internal operations remain undisclosed or invisible to users and other parties.
To validate and ensure high-quality synthetic data, it is imperative to compare synthetic and real data to measure accuracy and detect disparities.
At Fairgen, we allow users to validate our synthetic boosts through a straightforward parallel testing process. In this process, we demonstrate the reliability and statistical accuracy of synthetic sample boosts compared to real data kept on the side.
What are the leading synthetic data providers for research?
In a world driven by data, the foundational importance of high-quality data is paramount. We’ve already discussed the rise of synthetic data as a major player in consumer research. Now, let’s get the scoop on the companies that have navigated this landscape, establishing themselves as indispensable partners for research teams across diverse industries. Offering dependable, privacy-conscious solutions to fuel analytics, boost data collection and sample sizes, and facilitate various research initiatives, these are the leading synthetic data providers:
Fairgen
Fairgen is the first platform offering synthetic respondents with statistical guarantees for quantitative studies. Its flagship product, Fairboost, offers researchers and insights professionals the opportunity to analyze niche segments and overcome high margins of error using reliable generative AI technology. Its AI models are trained on each study separately, utilizing traditional statistics to learn trends and patterns on the data and matching it with modern GenAI technology to generate new, original and highly predictive, synthetic respondents. The technology is based on a rigorous scientific validation process and promises to double to triple, the effective sample size of niche groups.

Yabble
Yabble’s basis of AI tools includes Count, Gen, Summarize, and Augmented Data. Count allows for identifying improvement areas within the company’s strategies promoting ROI and driving the utility of consumer experiences. Gen serves as a conversational tool to answer any questions regarding the inputted data. Summarize generates in-depth analysis and accurate key findings of long-form data. Augmented Data boosts data analysis propelling further the capacity to interact with aforementioned tools.
Their newest innovation, Virtual Audiences, is an AI-driven personas powered feature utilizing the propriety Augmented Data model. This dynamic tool claims to eliminate the need for acquiring samples through fieldwork, as artificial personas are generated to offer insightful, relevant audience interactions. The employment of synthetic personas trained on the source data and other diverse sources offers researchers in-depth insights into market, or specific consumer, behaviors and trends.
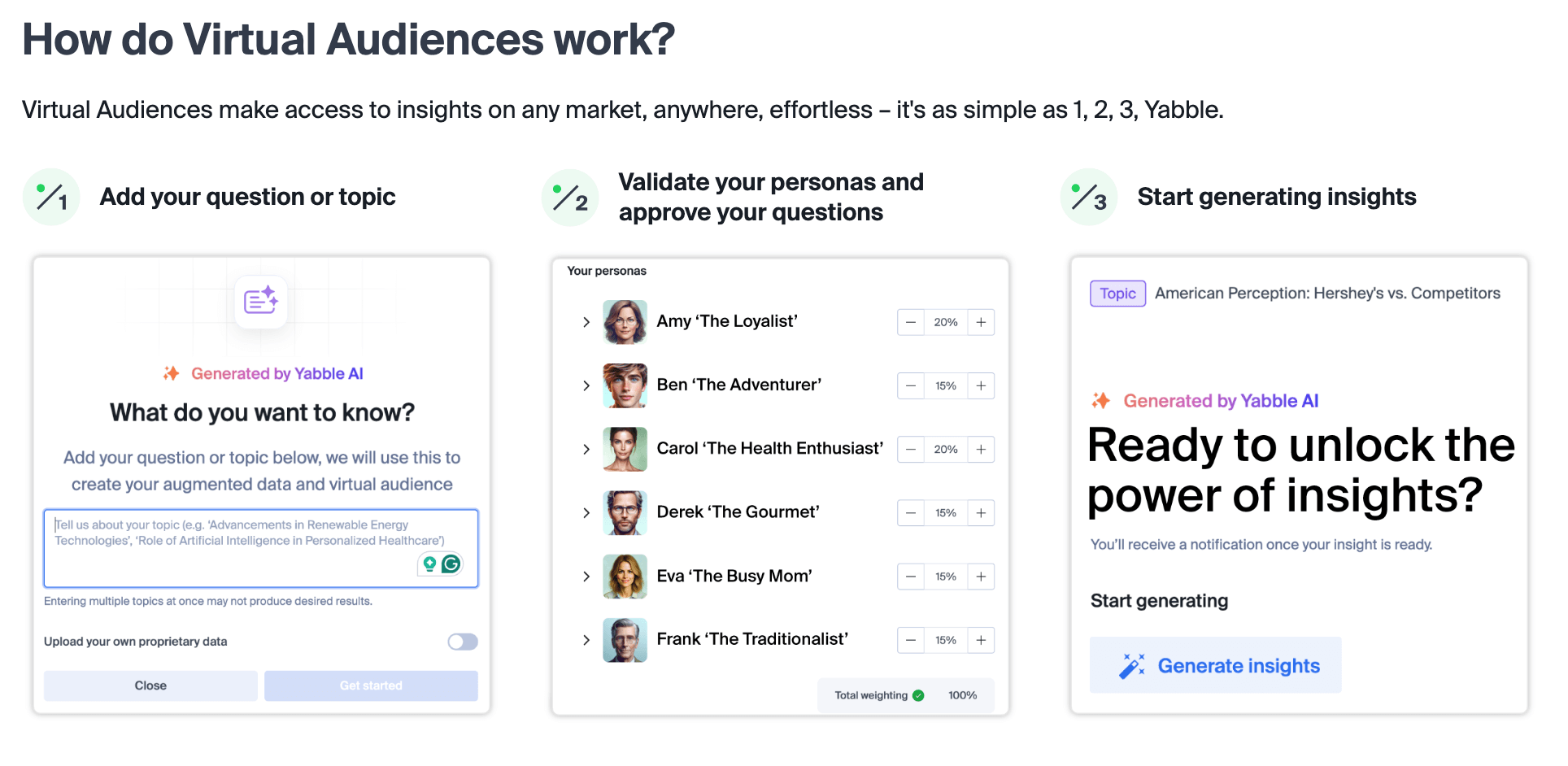
Synthetic Users
Synthetic Users is a platform trained on Large Language Models designed to test products and ideas using AI-generated personas.
Synthetic Users developed a conversational interface to employ surveys as tools for interacting with various personas. This simulation of real-world scenarios helps companies validate market behaviors, assess suitability, and gather feedback efficiently.

Roundtable
Roundtable is an AI-powered survey analysis platform that utilizes synthetic data to simulate responses and audiences. Through Alias, their Application Programming Interface, or API, users can distinctly explore and segment groups with survey questions. In addition, Roundtable offers a tool for flagging bot response patterns to automate cleaning and fraud detection within data. These features come together to better understand user data and gain confidence in niches needing action or further implementations.

Native AI
Native AI is an AI-powered consumer analytics platform that focuses on understanding and predicting preferences and behaviors utilizing NLP, or Natural Language Processing, features.
Through generative AI, qualitative and quantitative data points are analyzed to report on actionable recommendations and areas of improvement based on new market trends or fits. Insight features include dashboards, visualizations, reporting features, and automation of product tracking across the web.
Digital Twins, their propriety solution, creates interactive market research participants that emulate target customers. Users can collect predictive responses through the Twins feature leveraging this match in panel criteria to attain insight on product preferences. Interactive dashboard insights monitoring company trends and competition.

OpinioAI
OpinioAI uses AI language models to streamline access to pertinent insights, data, and opinions without depending on real-world traditional methods. Through synthetic sampling and data generation, OpinioAI offers users a comprehensive understanding of their market segments.
OpinioAI includes features such as Persona Builder, Ask Away, Analyze, and Evaluate Messaging.
- Persona Builder offers users the space to build detailed buyer personas that illuminate specific interests and demographics all relevant to the focus questions.
- The next step falls under Ask Away, in which these personas respond to specific questions based on predefined parameters of your research.
- The Analyze feature utilizes AI to as the name states, analyze and process inputted datasets, research publications, or reports.
- Evaluate Messaging touches base with the generated artificial personas to gain insightful feedback and assess positioning statements.
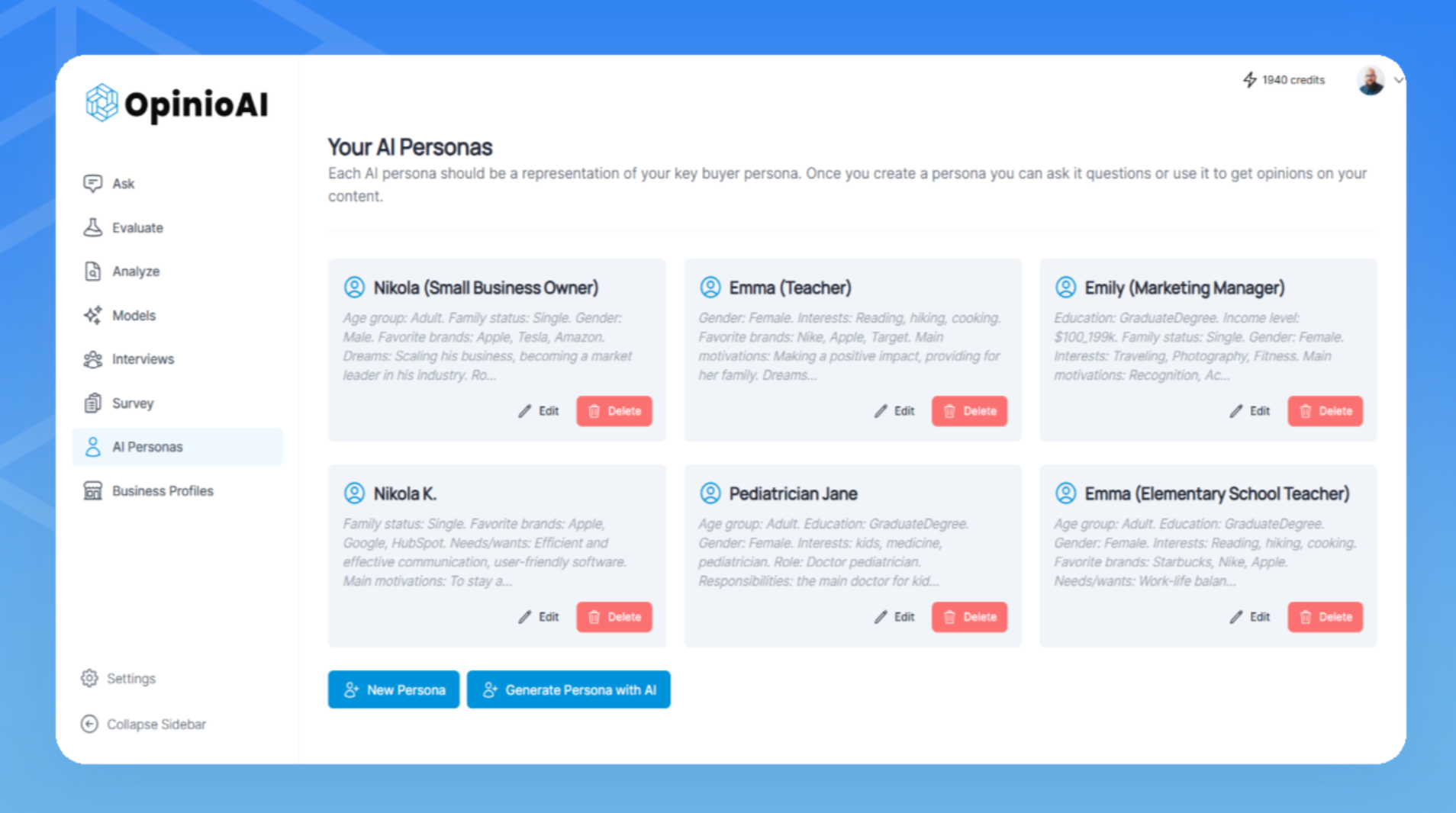
Concluding Remarks
The undeniable potential of synthetic data within the sphere of market research is apparent. As researchers and businesses dive deeper into this innovative technology, it becomes increasingly clear that it holds the keys to unprecedented levels of efficacy, creativity, and competitiveness.
According to Greenbook, 76% of participants from the research industry believe that generative AI technology will improve their organization’s competitiveness in the marketplace.
Here at Fairgen, we believe that the market is ready to learn, adapt, and reliably deploy new technology for faster and deeper insights. We continue in our mission to accelerate time-to-insights with responsibility. Come join us at the forefront of the consumer research industry!