Discover how Fairgen evaluates the validity of synthetic respondents to revolutionize market research with reliable granular insights.
Introduction
Since its inception, Fairgen’s purpose has been to safeguard the reliability of data for informed business decision-making. After talking to various leaders in the insights sector, we identified a widespread challenge: researchers can’t deliver granular insights at the pace modern business requires due to high data collection costs.
These niche segments can range from age groups (e.g., GenZ), product consumers (e.g., L’Oréal Kérastase customers), or even company-specific criteria (e.g., company size or role). Gathering enough data from these groups is typically expensive because the price per respondent is inversely proportional to the size of the segment, i.e., the smaller the segment, the more expensive the respondent. However, getting reliable insights is directly correlated with having enough data to back them up.
With years of experience in the field of synthetic data, Fairgen’s founding team decided to tackle this problem using a Generative AI-based approach. The main question was whether we could increase the number of respondents from these smaller segments by extrapolating what we had learned from similar segments and patterns in the rest of the data. When we first pitched this idea to research companies, pretty much every company but one (IFOP) told us there would never be a world where we would be able to use synthetically generated respondents to complete surveys.
We believe the timing is right for a third revolution in data collection for research.
The first revolution began in the 1930s when Gallup showed the value of leveraging consumer feedback, gathered via phone sampling, for business decisions. The second wave emerged in the early 2000s with the realization that online sampling was as reliable as traditional methods. We see the next evolutionary step as integrating real samples with AI-generated responses.
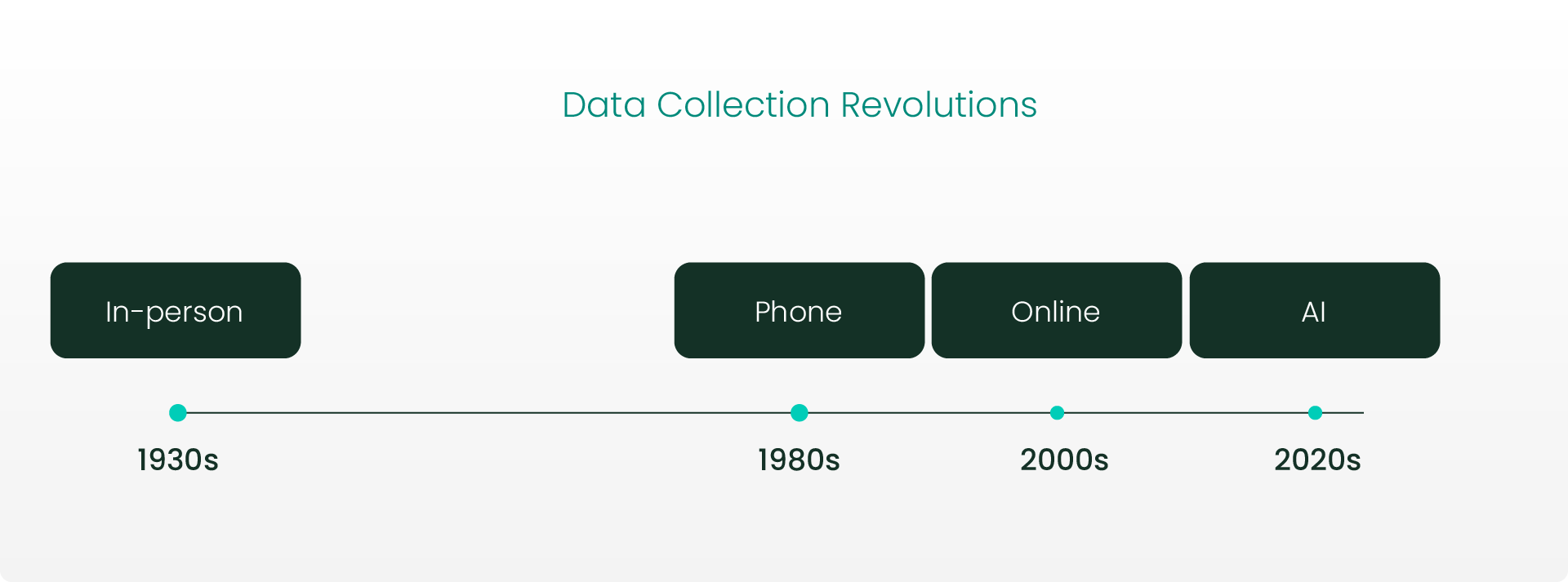
Despite skepticism, we were confident that this advancement was inevitable, provided we could demonstrate its effectiveness, mirroring Gallup’s breakthroughs and the validation of online polling.
Furthermore, the well-known variability in the quality of survey respondents, characterized by issues like respondent fatigue and the presence of bots, or disengaged participants, gaming the system for incentives, highlights the pressing need for innovation in data collection methodologies. These challenges, which directly impact the reliability and accuracy of research findings, make a compelling case for adopting novel approaches, such as synthetic data generation, to ensure higher-quality insights.
Fast-forward a year and a half, and synthetic data has become a cornerstone topic in market research, with Fairgen leading the conversation and collaboration in this space. In this article, we explain our progress thus far and the strength of our models. We also explore our rigorous methodology validation process and clarify how we ensure the reliability and accuracy of our synthetic data.
As we continue to innovate and push the boundaries of synthetic data, we invite you to join us on this journey to modernize market research and unlock new areas of insight.
The problem
Consider a scenario where market research company X gathers data from 1,000 respondents, but only 50 are from a specific group of interest. A sample size of 50 is often too small to yield reliable insights, resulting in overly broad confidence intervals that undermine decision-making.
Company X may have to return to the field and find 50 more respondents in this group to strengthen the validity of these insights. However, the cost and time to do so is high. Our solution aims to double the effective sample size for any segment constituting less than 15% of the total base, thereby achieving the same level of precision as if the data were physically collected. This “doubling” means that our enhanced base would produce results with the same margin of error as physically collecting twice as much data, providing more reliable insights without the additional cost and time.
Scientific Validation
In this section, we show that, on average, Fairgen’s technology provides the same amount of error reduction as if we had 2 to 4 times more data to begin with.
We rigorously evaluated Fairgen's synthetic samples, hereafter referred to as fairsets, both qualitatively and quantitatively. Below, we provide a taste of our evaluation. Let's dive in.
How do we measure performance?
To make accurate inferences from data, we want it to correspond to the true population distribution as much as possible. Naturally, the data sampled when polling is a noisy estimate of the population distribution. The more samples we have, the more accurate our estimate of the population distribution. We measure the error of our data as the distance from a large holdout set, the latter serving as a proxy for the actual, unmeasurable population distribution. We refer to this holdout set as our ground truth. We then compare this error to a sequence of errors obtained from training sets of different lengths, which serve as a yardstick to indicate the value of the synthetic sample set in terms of real dataset size. The factor by which we must increase (or decrease) the sample size to achieve the same error as a given set of samples is called Effective Sample Size (ESS). Hence, a subset with the same error achieved by the original sample has an ESS of 1.
Spoiler: On average, the ESS for Fairgen is greater than 2.5.

Measuring the ESS for Fairgen
We average the ESS for each segment of the data to get the ESS of a dataset.
For our evaluation, we selected multiple columns containing demographic information (e.g., age, gender, etc.) to define the segments. Each segment is a subset of the training data for which a subset of the demographic columns take on a specific set of values (e.g., Women between the ages of 30 and 50). To constrain the total number of segments used in the evaluation, we limit our evaluation to segments defined by one or two demographic columns.
Segments with extremely low support in the training data cannot be boosted. On the other hand, very large segments likely provide a good estimate of the ground truth and, therefore, do not require more respondents. Therefore, we only boost segments that comprise between 1% and 15% of the data. This intermediate range of segment size corresponds to situations in which a researcher would need to collect additional data.
Performance for Wave 112 from Pew Research
Fairgen's synthetic samples more closely resemble the ground truth distribution than the original training sample. We show this using a social media usage poll (Wave 112) conducted by the Pew Research Center, with 12,147 responses. The demographics selected for our evaluation on this dataset were race, religion, marital status, party affiliation, education level, and whether the respondent uses social media.
The mean ESS for the Wave 112 dataset is 2.79, equivalent to increasing the number of respondents from 1,000 to 2,790.
In the plots below, we compare the distributions of the training sample, the fairset, and the ground truth. We show our results in a grid where each column corresponds to a specific segment, and each row corresponds to a specific question (e.g., the top right sub-plot shows the distributions of the question “Which do you prefer for getting the news?” for the segment of high school graduates with the marital status “Divorced”). As seen below, the fairset often better approximates the ground truth distribution.
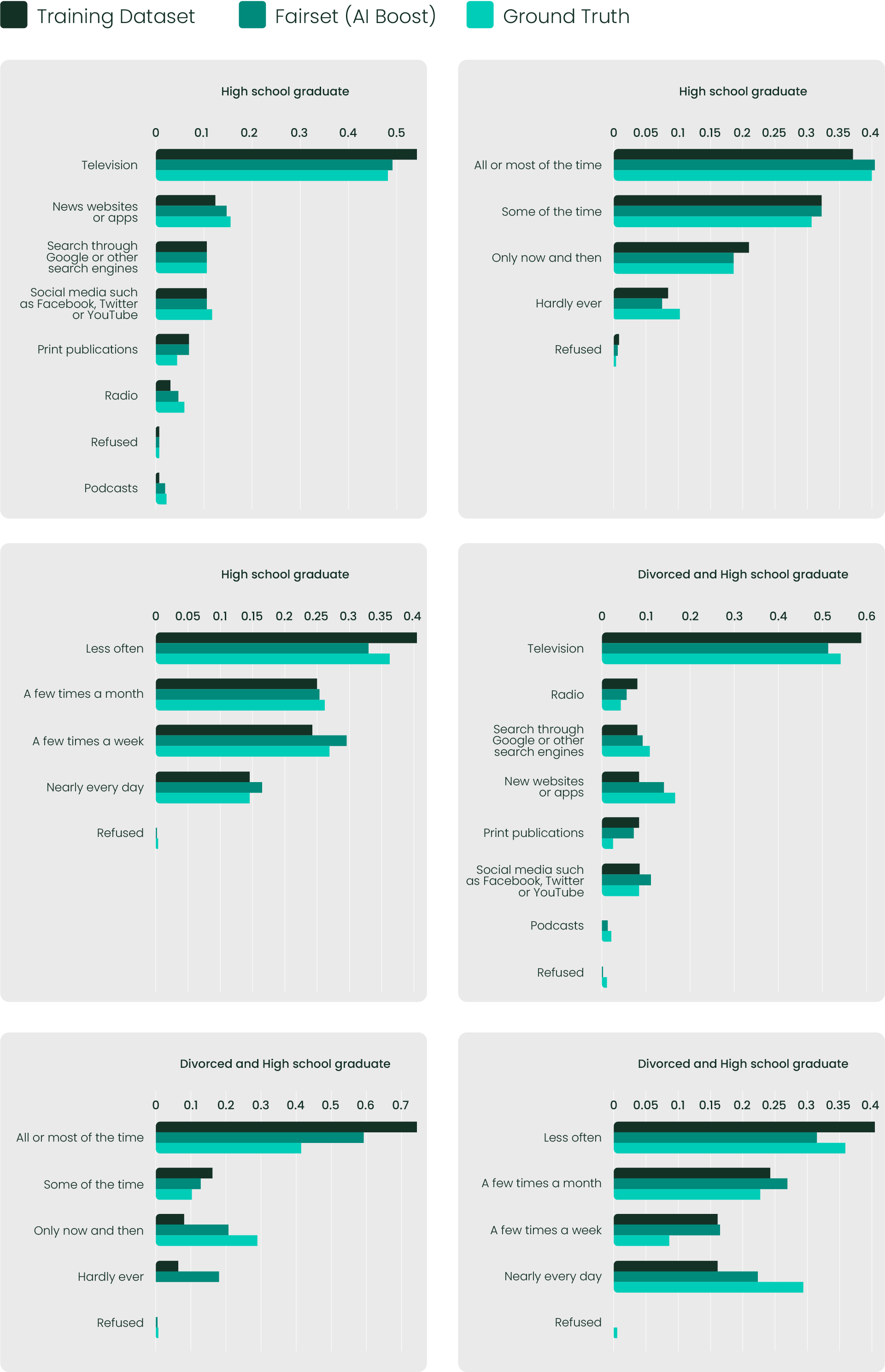
Performance on Pew corpus
For a robust quantitative evaluation, we use all of the publicly available datasets from the Pew Research Center’s American Trends Panel with at least 10,000 samples and include multiple demographic columns. This dataset corpus highly represents the market research use cases in which Fairgen can provide significant value. In total, we include 40 datasets in our evaluation.
Fairgen’s technology works with a wide range of column types. However, some columns should not (e.g., constant value columns) or cannot be boosted (e.g., ID columns). Fairgen, therefore, applies customized logic to these columns. As is often the case with survey data, the vast majority of the columns in the datasets are categorical.
The demographics that define segments were manually selected based on the datasets’ documentation. We selected between three and seven demographic columns for each dataset. Some examples of demographic columns are age, race, religion, gender, and party affiliation. In our evaluation, we used 7,316 segments with sizes in the range [10,150] with a mean of 48.26 and a standard deviation of 37.93. A histogram of the segment sizes is shown below.
.png)
All of the datasets included between 10,000 and 14,500 samples. A box plot of the number of samples in all the datasets is provided below. The majority of the datasets have between 10,000 and 11,000 samples.
.png)
Generating a fairset depends on the training data. We take this into account by averaging over 3 random training/ground truth partitions of each dataset. In each partition, we allocate 1,000 samples for training; the remaining samples serve as the ground truth. This enables us to estimate both the expected ESS and its variance.
The mean ESS for all of the datasets was 2.855, with a standard deviation of 0.156. The mean ESS for each dataset was in the range of [1.85, 3.33]. Below, we show the relationship between ESS and the segment size before boosting with synthetic data. We show the mean ESS as a dark line, and the interquartile region is shaded in the background. Smoothing with a rolling window of size ten was applied to data for visualization purposes. Smaller segments benefit the most from Fairgen. The smallest segments have an ESS of around 3.5, which represents a 3.5x increase in the quality of the estimation of the ground truth distribution! As the number of training samples increases, the ESS factor decreases due to the training data better approximating the ground truth distribution.
Overall, Fairgen excels in low-data scenarios where acquiring more respondents is the most difficult.
.png)
Conclusion
In this article, we have detailed our methodology for evaluating the validity of our synthetic respondents.
Under the guidelines described here, we achieve an average boost factor of 3x, which means that our data allows us to zoom in to levels of granularity that would have required collecting three times more data to begin with.
Practically, this means we can drive insights at an unprecedented depth, hence potentially helping to improve processes in areas such as product development, user testing, opinion studies, brand equity, engagement, and more. We recommend replicating these experiments using your own data to observe the boost factor from your own perspectives.
The momentum is growing as more industry leaders recognize and leverage the advantages of synthetic respondents. In a world where data insights drive business decisions, adopting our validated methodology is an opportunity to stay at the forefront of innovation. We encourage you to lead this charge. By embracing and advocating for this technology now, you position your organization not only as an adopter but as a leader in the next phase of market research evolution.
Join us in reshaping the landscape, setting new standards, and unlocking unparalleled insights that propel us all forward.